Data Science in der Finanzökonomik
Die Forschungsgruppe „Data Science in der Finanzökonomik“ konzentriert sich auf die Entwicklung und Anwendung neuartiger Methoden aus dem Bereich Data Science und Künstliche Intelligenz im Kontext der Finanzökonomik. Diese Methoden dienen dazu, aus unstrukturierten Daten – wie Textdaten, Satellitenbildern oder Webscraping – ökonomische Indikatoren zu generieren. Diese Indikatoren werden anschließend in ökonometrischen Analysen eingesetzt, um zentrale Fragestellungen der Finanzökonomik zu untersuchen. Forschungsprojekte der Gruppe nutzen solche Indikatoren unter anderem zur Analyse von:
Informationsfriktionen auf Finanzmärkten, etwa im Hinblick auf die Qualität von Unternehmensberichten und Corporate Governance.
Kreditvergabeverhalten von Banken und Risikoverschiebungen auf Immobilienmärkten als Reaktion auf makroprudenzielle Regulierung.
Die Anpassung von Märkten an den Klimawandel und deren Grenzen.
Die Forschungsgruppe ist zudem an der Entwicklung des European Real Estate Index (EREI) des IWH beteiligt, der europäische Immobilienmärkte systematisch erfasst. Der EREI enthält Daten zu Angebotspreisen, Angebotstiefe (Anzahl der Inserate) und Liquidität (Verweildauer). Harmonisiert auf der NUTS-3-Regionsebene ermöglicht der Index konsistente länderübergreifende Vergleiche sowie räumliche Analysen für Wissenschaft, Politik und Öffentlichkeit.
Forschungscluster
Finanzresilienz und RegulierungIhr Kontakt

- Abteilung Finanzmärkte
Referierte Publikationen
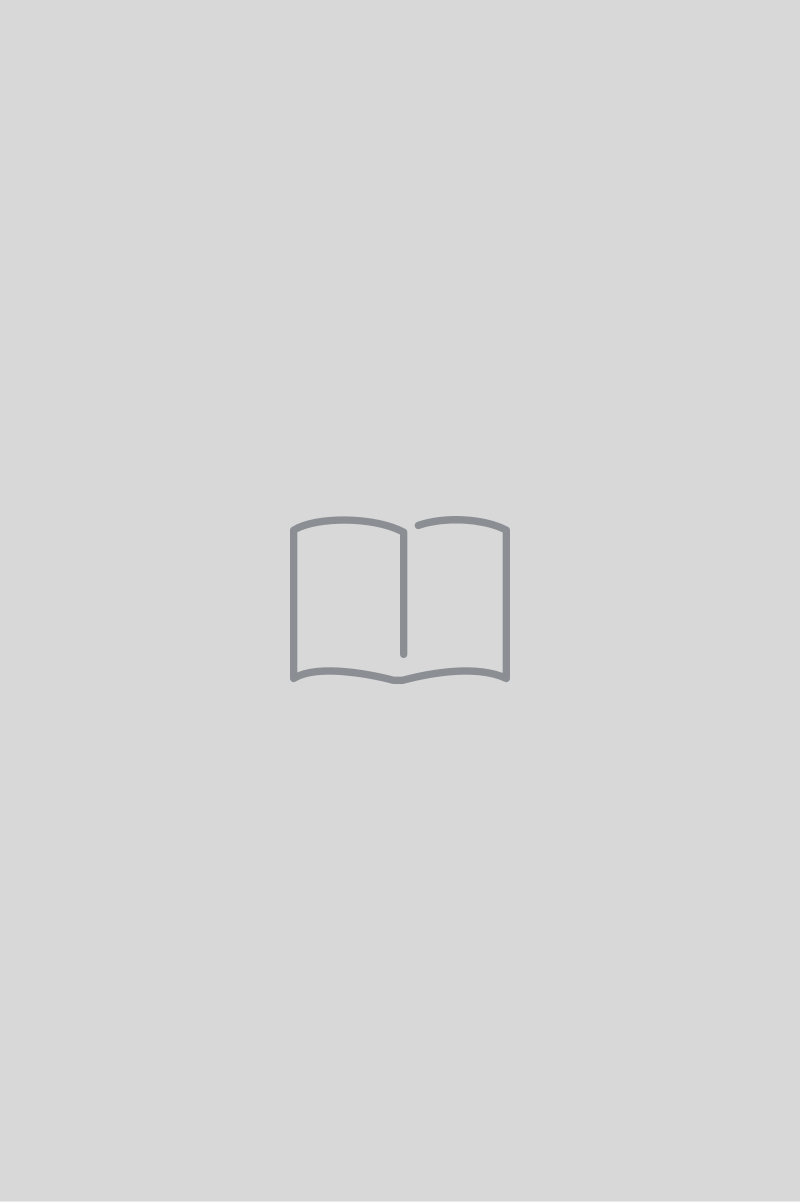
Understanding CSR Champions: A Machine Learning Approach
in: Annals of Operations Research, im Erscheinen
Abstract
<p>In this paper, we study champions of corporate social responsibility (CSR) performance among the U.S. publicly traded firms and their common characteristics by utilizing machine learning algorithms to identify predictors of firms’ CSR activity. We contribute to the CSR and leadership determinants literature by introducing the first comprehensive framework for analyzing the factors associated with corporate engagement with socially responsible behaviors by grouping all relevant predictors into four broad categories: corporate governance, managerial incentives, leadership, and firm characteristics. We find that strong corporate governance characteristics, as manifested in board member heterogeneity and managerial incentives, are the top predictors of CSR performance. Our results suggest policy implications for providing incentives and fostering characteristics conducive to firms “doing good.”</p>
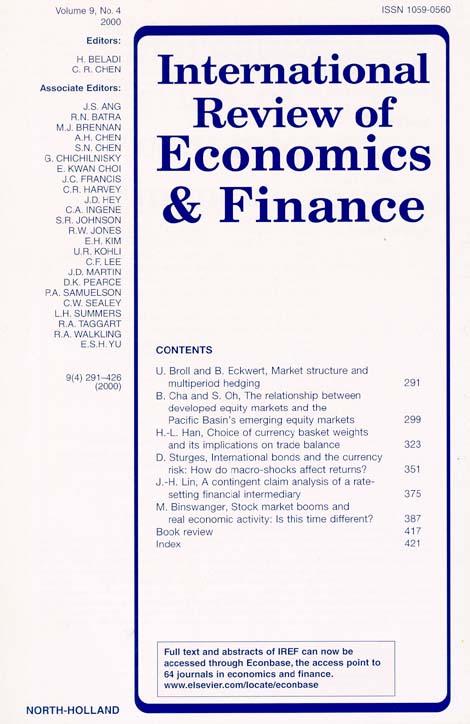
Search Symbols, Trading Performance, and Investor Participation
in: International Review of Economics and Finance, April 2024
Abstract
<p>We investigate the relationships among search symbols, trading performance, and investor participation. We use two specific datasets from Google Trends’ search volume index. The search volume by number ticker significantly predicts high returns and high investor participation when applied by active retail investors investing in large firms. This does not hold true for less active retail investors who use Chinese company name tickers as their search terms. Our results indicate that the heuristic usage of number tickers to search for company information helps active retail investors to obtain better trading performance compared with less active retail investors who use Chinese company name tickers.</p>
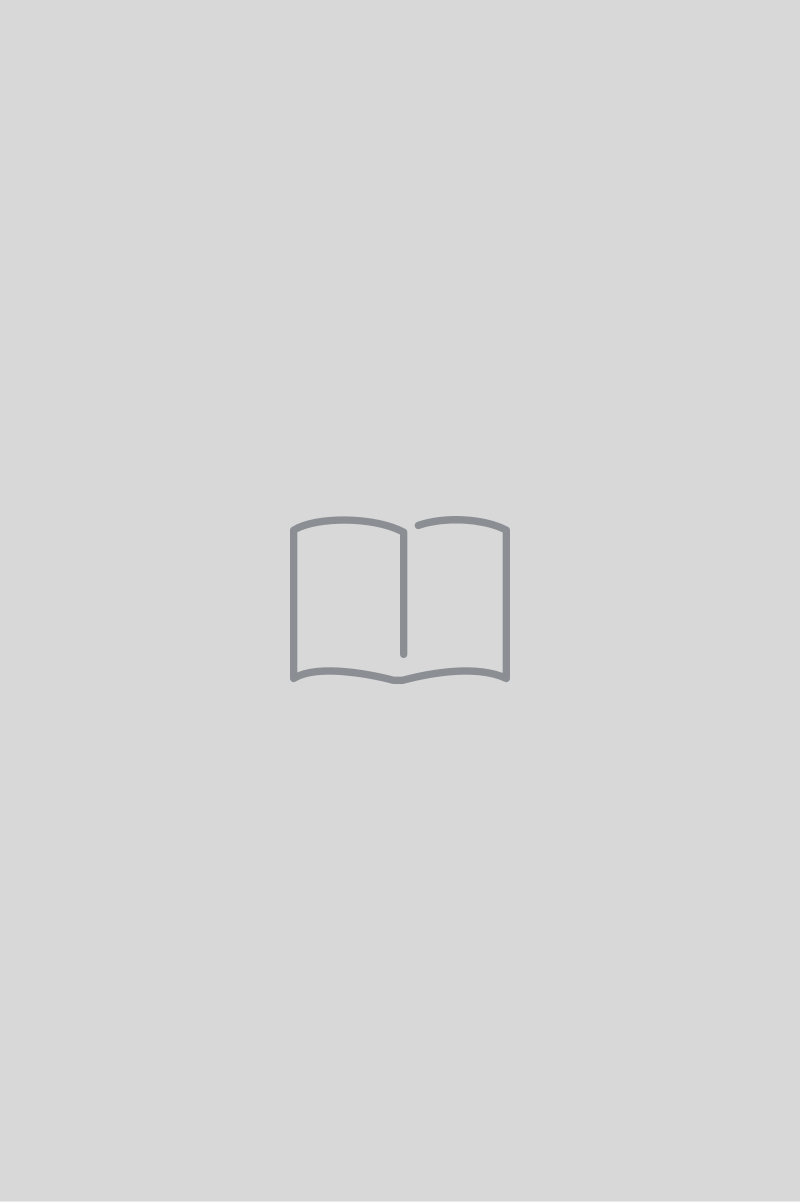
Asymmetric Reactions of Abnormal Audit Fees Jump to Credit Rating Changes
in: British Accounting Review, Nr. 2, 2024
Abstract
Considering the inherent stickiness of abnormal audit fees, our study contributes to the literature by decomposing abnormal audit fees into a jump component and long-run sticky component. We investigate whether and how changes in credit ratings asymmetrically affect the jump component of abnormal audit fees. We document a positive association between rating downgrades and the jump component. We find that heightened bankruptcy risk and misstatement risk are the mechanisms that drive this relationship. Further analysis shows that firms experiencing rating downgrades are more likely to receive a going concern opinion and experience longer audit report lags. Taken together, our findings provide direct evidence that credit ratings are significantly associated with abnormal audit fees, particularly with the jump component. Given the serial correlation of abnormal audit fees, our study sheds light on the importance of disaggregation of the abnormal audit fee residuals into the jump and long-run sticky components.
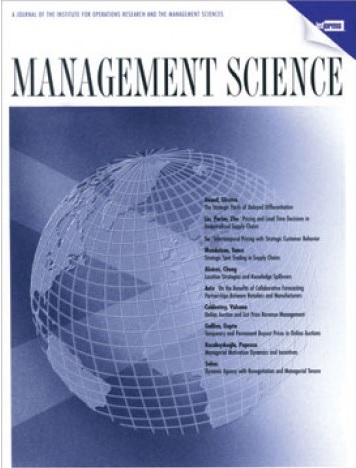
"Let Me Get Back to You" — A Machine Learning Approach to Measuring NonAnswers
in: Management Science, Nr. 10, 2023
Abstract
Using a supervised machine learning framework on a large training set of questions and answers, we identify 1,364 trigrams that signal nonanswers in earnings call questions and answers (Q&A). We show that this glossary has economic relevance by applying it to contemporaneous stock market reactions after earnings calls. Our findings suggest that obstructing the flow of information leads to significantly lower cumulative abnormal stock returns and higher implied volatility. As both our method and glossary are free of financial context, we believe that the measure is applicable to other fields with a Q&A setup outside the contextual domain of financial earnings conference calls.
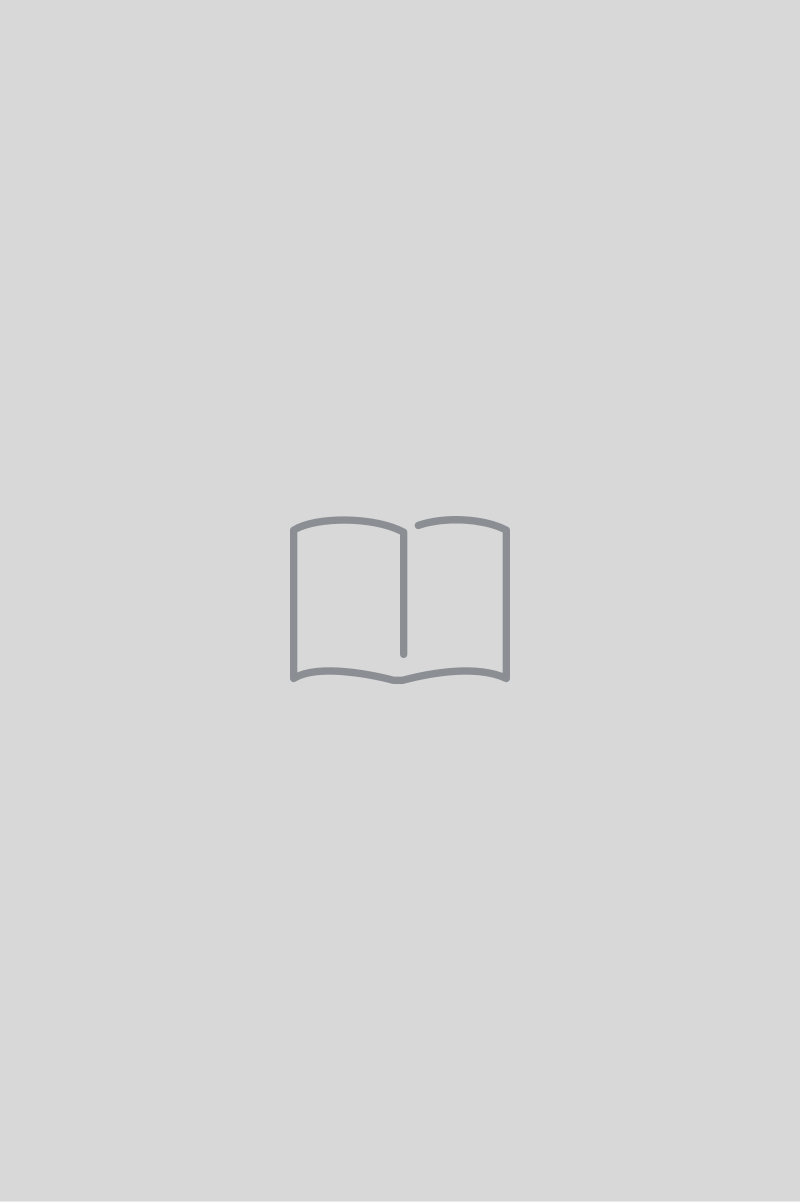
Exploring Accounting Research Topic Evolution: An Unsupervised Machine Learning Approach
in: Journal of International Accounting Research, Nr. 3, 2023
Abstract
This study explores the evolution of accounting research by utilizing an unsupervised machine learning approach. We aim to identify the latent topics of accounting from the 1980s up to 2018, the dynamics and emerging topics of accounting research, and the economic reasons behind those changes. First, based on 23,220 articles from 46 accounting journals, we identify 55 topics using the latent Dirichlet allocation model. To illustrate the connection between topics, we use HistCite to generate a citation map along a timeline. The citation clusters demonstrate the “tribalism” phenomenon in accounting research. We then implement the dynamic topic model to reveal the dynamics of topics to show changes in accounting research. The emerging research trends are identified from the topic analytics. We further explore the economic reasons and in-depth insights into the topic evolution, indicating the economic development embeddedness nature of accounting research.
Arbeitspapiere
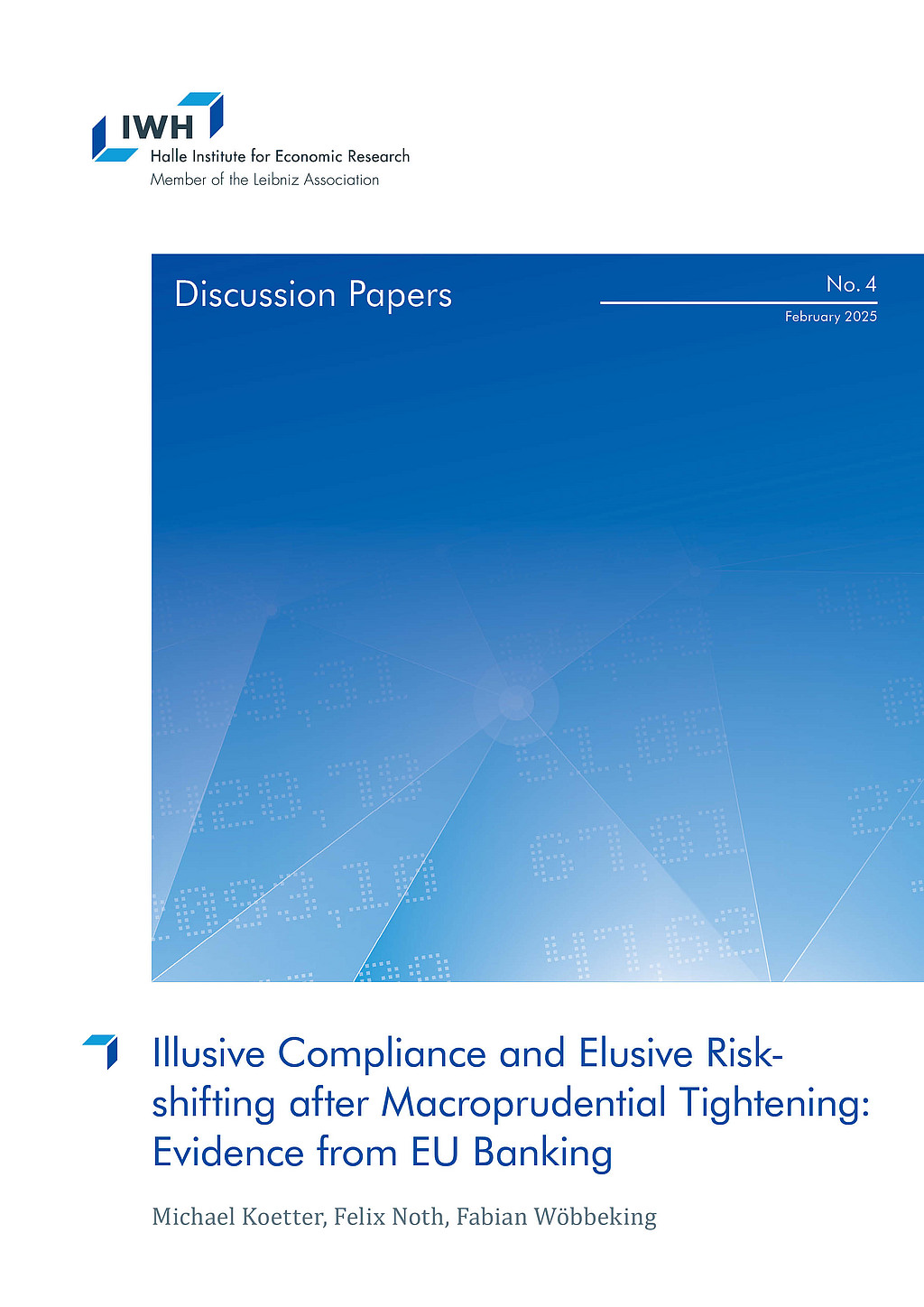
Illusive Compliance and Elusive Risk-shifting after Macroprudential Tightening: Evidence from EU Banking
in: IWH Discussion Papers, Nr. 4, 2025
Abstract
<p>We study whether and how EU banks comply with tighter macroprudential policy (MPP). Observing contractual details for more than one million securitized loans, we document an elusive risk-shifting response by EU banks in reaction to tighter loan-to-value (LTV) restrictions between 2009 and 2022. Our staggered difference-in-differences reveals that banks respond to these MPP measures at the portfolio level by issuing new loans after LTV shocks that are smaller, have shorter maturities, and show a higher collateral valuation while holding constant interest rates. Instead of contracting aggregate lending as intended by tighter MPP, banks increase the number and total volume of newly issued loans. Importantly, new loans finance especially properties in less liquid markets identified by a new European Real Estate Index (EREI), which we interpret as a novel, elusive form of risk-shifting.</p>
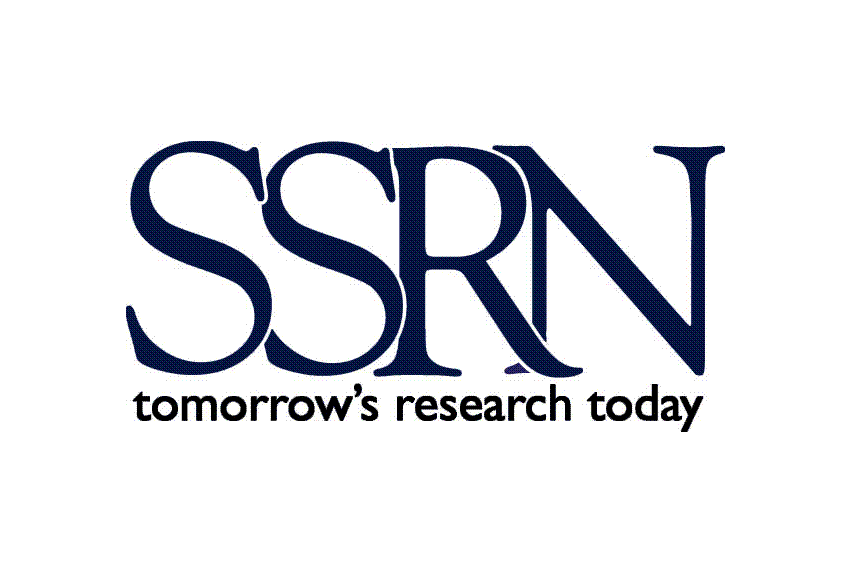
How to Talk Down Your Stock Performance
in: SSRN Discussion Papers, 2020
Abstract
We process the natural language of verbal firm disclosures in order to study the use of context specific language or jargon and its impact on financial performance. We observe that, within the Q&A of earnings conference calls, managers use less jargon in responses to tougher questions, and after a quarter of bad economic success. Moreover, markets interpret the lack of precise information as a bad signal: we find lower cumulative abnormal returns and a higher implied volatility following earnings calls where managers use less jargon. These results support the argument that context specific language or jargon helps to efficiently and precisely transfer information.