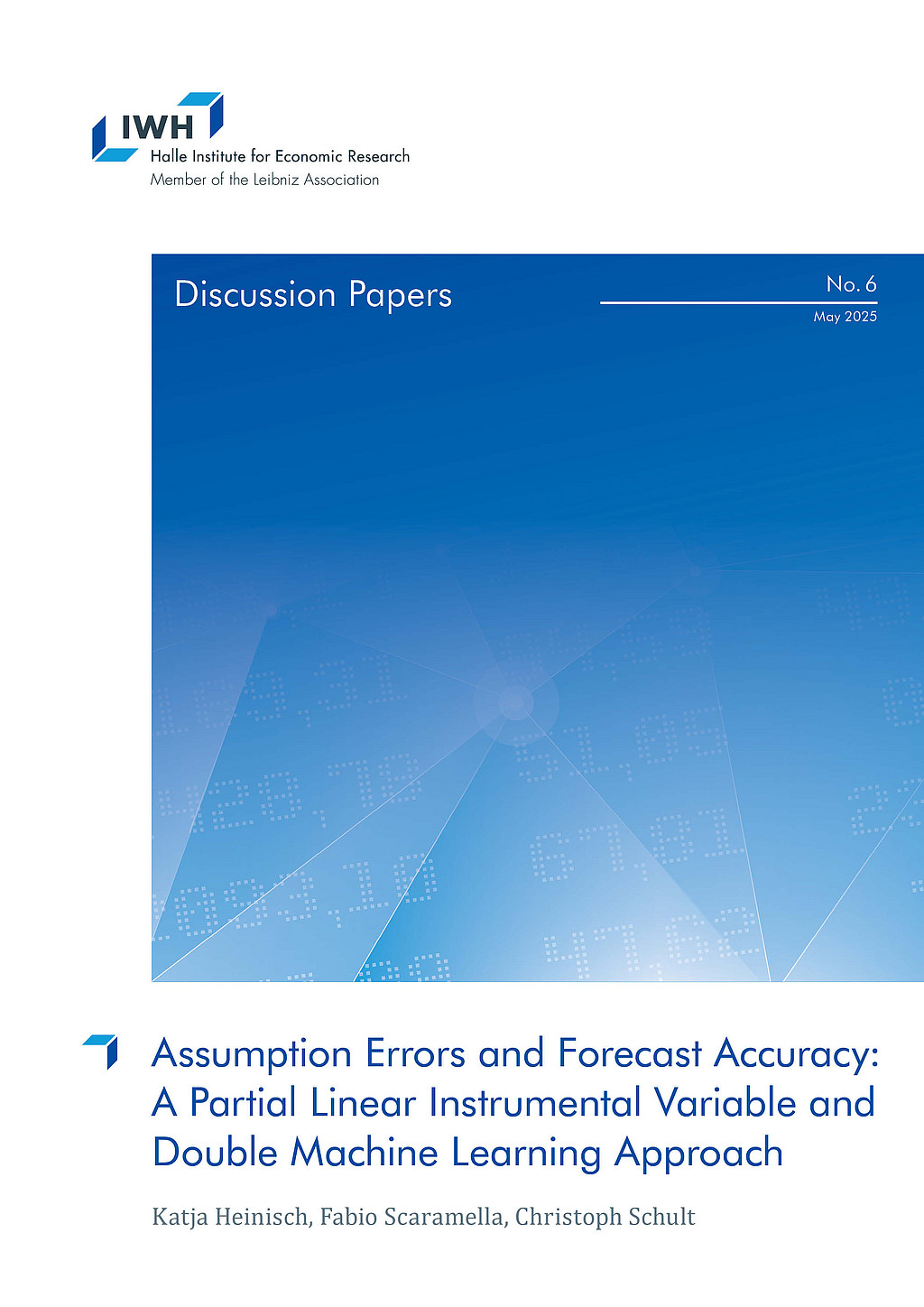
Assumption Errors and Forecast Accuracy: A Partial Linear Instrumental Variable and Double Machine Learning Approach
Accurate macroeconomic forecasts are essential for effective policy decisions, yet their precision depends on the accuracy of the underlying assumptions. This paper examines the extent to which assumption errors affect forecast accuracy, introducing the average squared assumption error (ASAE) as a valid instrument to address endogeneity. Using double/debiased machine learning (DML) techniques and partial linear instrumental variable (PLIV) models, we analyze GDP growth forecasts for Germany, conditioning on key exogenous variables such as oil price, exchange rate, and world trade. We find that traditional ordinary least squares (OLS) techniques systematically underestimate the influence of assumption errors, particularly with respect to world trade, while DML effectively mitigates endogeneity, reduces multicollinearity, and captures nonlinearities in the data. However, the effect of oil price assumption errors on GDP forecast errors remains ambiguous. These results underscore the importance of advanced econometric tools to improve the evaluation of macroeconomic forecasts.
19. Mai 2025
https://doi.org/10.18717/dprpy3-ff77