Data Science in Financial Economics
This group focuses on developing and applying novel data science tools in the area of financial economics. One particular focus is on applying data science methods to generate economic indicators from unstructured data, such as textual and imagery data or web scraping.
Research Cluster
Financial Resilience and RegulationYour contact
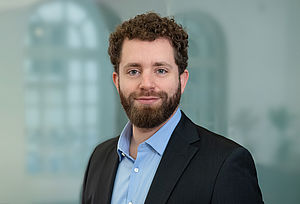
Mitglied - Department Financial Markets
Refereed Publications
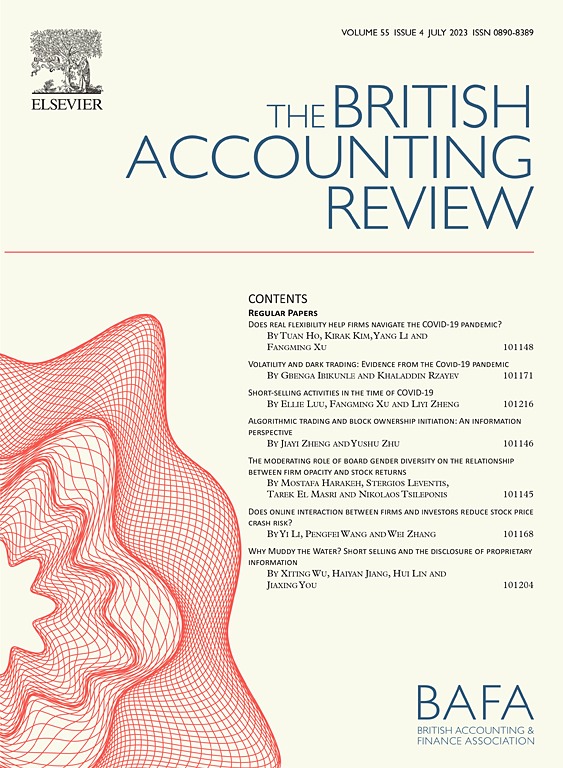
Asymmetric Reactions of Abnormal Audit Fees Jump to Credit Rating Changes
in: British Accounting Review, forthcoming
Abstract
Considering the inherent stickiness of abnormal audit fees, our study contributes to the literature by decomposing abnormal audit fees into a jump component and long-run sticky component. We investigate whether and how changes in credit ratings asymmetrically affect the jump component of abnormal audit fees. We document a positive association between rating downgrades and the jump component. We find that heightened bankruptcy risk and misstatement risk are the mechanisms that drive this relationship. Further analysis shows that firms experiencing rating downgrades are more likely to receive a going concern opinion and experience longer audit report lags. Taken together, our findings provide direct evidence that credit ratings are significantly associated with abnormal audit fees, particularly with the jump component. Given the serial correlation of abnormal audit fees, our study sheds light on the importance of disaggregation of the abnormal audit fee residuals into the jump and long-run sticky components.
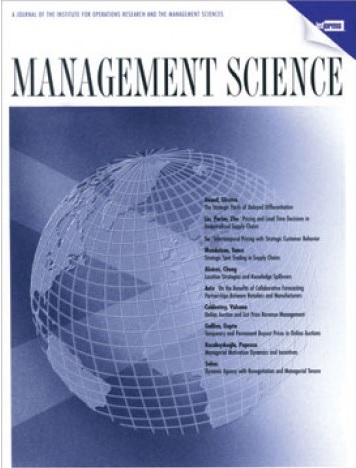
"Let Me Get Back to You" — A Machine Learning Approach to Measuring NonAnswers
in: Management Science, No. 10, 2023
Abstract
Using a supervised machine learning framework on a large training set of questions and answers, we identify 1,364 trigrams that signal nonanswers in earnings call questions and answers (Q&A). We show that this glossary has economic relevance by applying it to contemporaneous stock market reactions after earnings calls. Our findings suggest that obstructing the flow of information leads to significantly lower cumulative abnormal stock returns and higher implied volatility. As both our method and glossary are free of financial context, we believe that the measure is applicable to other fields with a Q&A setup outside the contextual domain of financial earnings conference calls.

Exploring Accounting Research Topic Evolution: An Unsupervised Machine Learning Approach
in: Journal of International Accounting Research, No. 3, 2023
Abstract
This study explores the evolution of accounting research by utilizing an unsupervised machine learning approach. We aim to identify the latent topics of accounting from the 1980s up to 2018, the dynamics and emerging topics of accounting research, and the economic reasons behind those changes. First, based on 23,220 articles from 46 accounting journals, we identify 55 topics using the latent Dirichlet allocation model. To illustrate the connection between topics, we use HistCite to generate a citation map along a timeline. The citation clusters demonstrate the “tribalism” phenomenon in accounting research. We then implement the dynamic topic model to reveal the dynamics of topics to show changes in accounting research. The emerging research trends are identified from the topic analytics. We further explore the economic reasons and in-depth insights into the topic evolution, indicating the economic development embeddedness nature of accounting research.
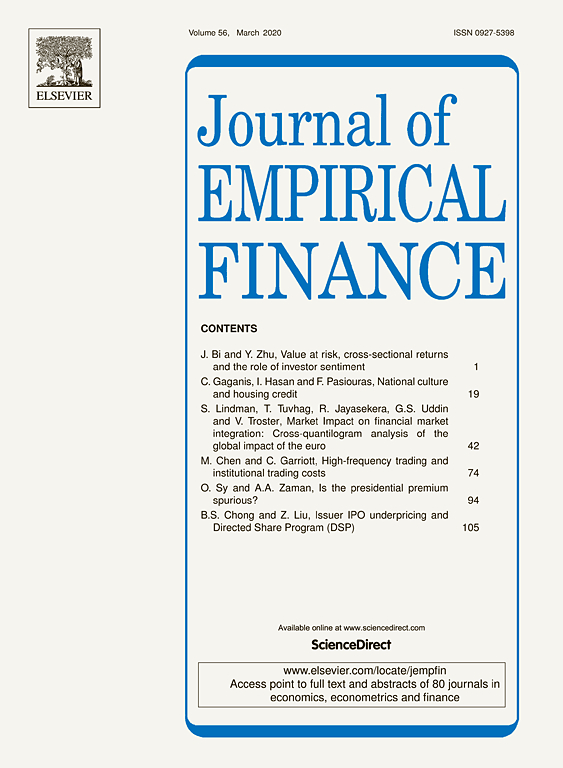
Herding Behavior and Systemic Risk in Global Stock Markets
in: Journal of Empirical Finance, September 2023
Abstract
This paper provides new evidence of herding due to non- and fundamental information in global equity markets. Using quantile regressions applied to daily data for 33 countries, we investigate herding during the Eurozone crisis, China’s market crash in 2015–2016, in the aftermath of the Brexit vote and during the Covid-19 Pandemic. We find significant evidence of herding driven by non-fundamental information in case of negative tail market conditions for most countries. This study also investigates the relationship between herding and systemic risk, suggesting that herding due to fundamentals increases when systemic risk increases more than when driven by non-fundamentals. Granger causality tests and Johansen’s vector error-correction model provide solid empirical evidence of a strong interrelationship between herding and systemic risk, entailing that herding behavior may be an ex-ante aspect of systemic risk, with a more relevant role played by herding based on fundamental information in increasing systemic risk.
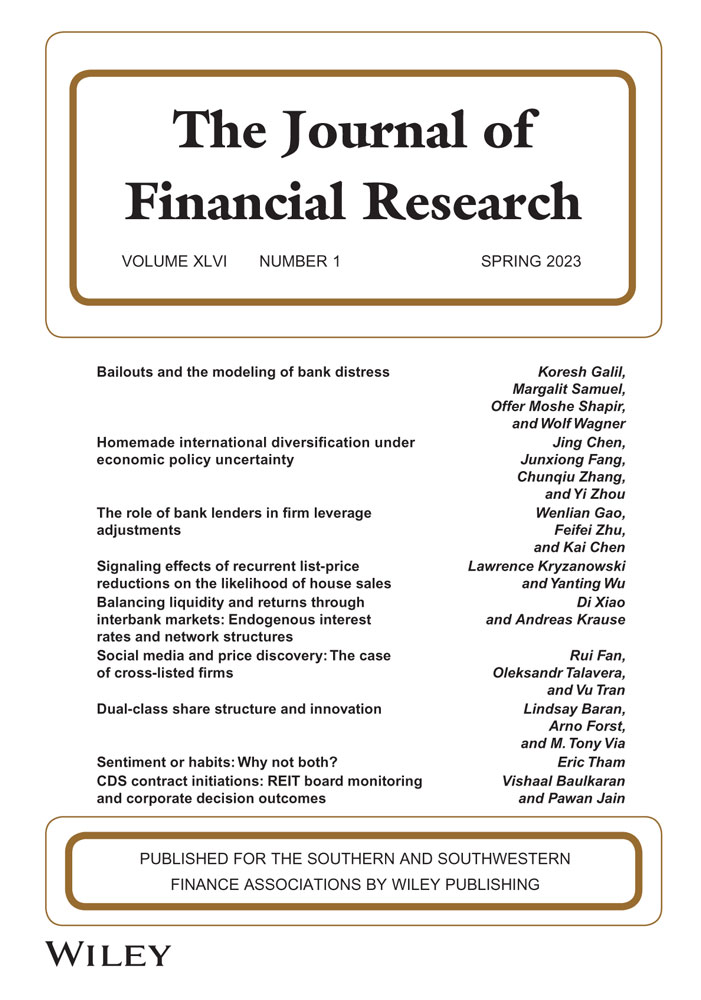
Market-implied Ratings and Their Divergence from Credit Ratings
in: Journal of Financial Research, No. 2, 2023
Abstract
In this article, we investigate the divergence between credit ratings (CRs) and Moody's market-implied ratings (MIRs). Our evidence shows that rating gaps provide incremental information to the market regarding issuers' default risk over CRs alone in the short horizon and outperform CRs over extended horizons. The predictive ability of rating gaps is greater for more opaque and volatile issuers. Such predictability was more pronounced during the 2008 financial crisis but weakened in the post-Dodd-Frank Act period. This finding is consistent with credit rating agencies' efforts to improve their performance when facing regulatory pressure. Moreover, our analysis identifies rating-gap signals that do (do not) lead to subsequent Moody's actions to place issuers on negative outlook and watchlists. We find that negative signals from MIR gaps have a real economic impact on issuers' fundamentals such as profitability, leverage, investment, and default risk, thus supporting the recovery-efforts hypothesis.
Working Papers
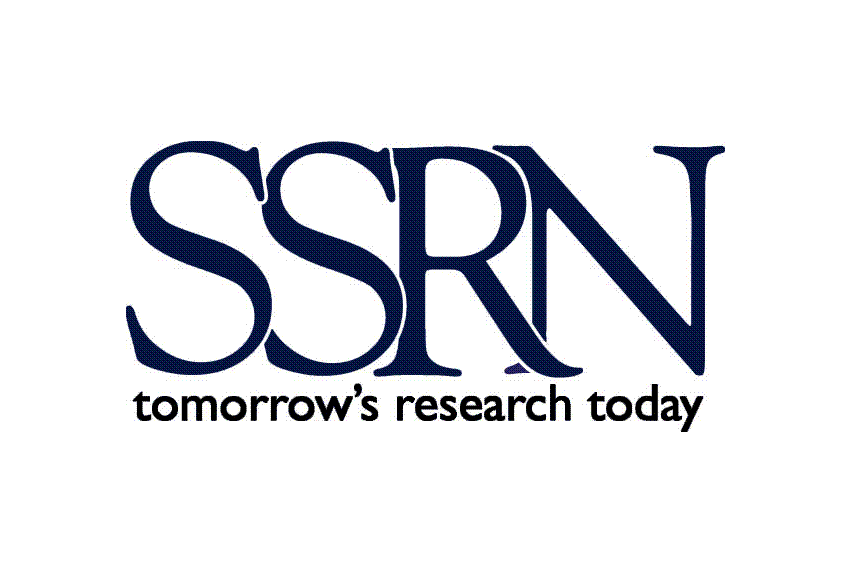
How to Talk Down Your Stock Performance
in: SSRN Discussion Papers, 2020
Abstract
We process the natural language of verbal firm disclosures in order to study the use of context specific language or jargon and its impact on financial performance. We observe that, within the Q&A of earnings conference calls, managers use less jargon in responses to tougher questions, and after a quarter of bad economic success. Moreover, markets interpret the lack of precise information as a bad signal: we find lower cumulative abnormal returns and a higher implied volatility following earnings calls where managers use less jargon. These results support the argument that context specific language or jargon helps to efficiently and precisely transfer information.