Data Science in der Finanzökonomik
Diese Gruppe konzentriert sich auf die Entwicklung und Anwendung neuartiger Data-Science-Tools im Bereich der Finanzökonomik. Ein Schwerpunkt ist die Anwendung von Data-Science-Methoden zur Gewinnung ökonomischer Indikatoren aus unstrukturierten Daten wie Text- und Bilddaten oder Web-Scraping.
Forschungscluster
Finanzresilienz und RegulierungIhr Kontakt
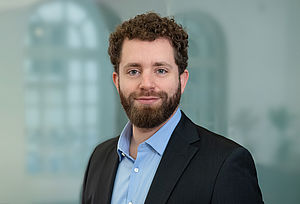
Mitglied - Abteilung Finanzmärkte
Referierte Publikationen
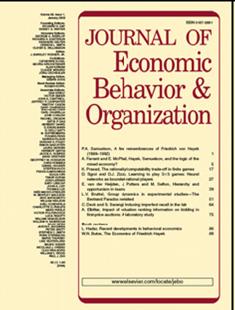
Correlation Scenarios and Correlation Stress Testing
in: Journal of Economic Behavior and Organization, January 2023
Abstract
We develop a general approach for stress testing correlations of financial asset portfolios. The correlation matrix of asset returns is specified in a parametric form, where correlations are represented as a function of risk factors, such as country and industry factors. A sparse factor structure linking assets and risk factors is built using Bayesian variable selection methods. Regular calibration yields a joint distribution of economically meaningful stress scenarios of the factors. As such, the method also lends itself as a reverse stress testing framework: using the Mahalanobis distance or Highest Density Regions (HDR) on the joint risk factor distribution allows to infer worst-case correlation scenarios. We give examples of stress tests on a large portfolio of European and North American stocks.
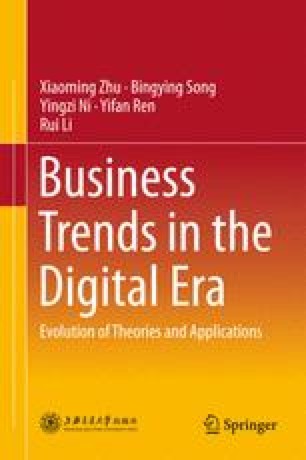
Cryptocurrency Volatility Markets
in: Digital Finance, Nr. 3, 2021
Abstract
By computing a volatility index (CVX) from cryptocurrency option prices, we analyze this market’s expectation of future volatility. Our method addresses the challenging liquidity environment of this young asset class and allows us to extract stable market implied volatilities. Two alternative methods are considered to compute volatilities from granular intra-day cryptocurrency options data, which spans over the COVID-19 pandemic period. CVX data therefore capture ‘normal’ market dynamics as well as distress and recovery periods. The methods yield two cointegrated index series, where the corresponding error correction model can be used as an indicator for market implied tail-risk. Comparing our CVX to existing volatility benchmarks for traditional asset classes, such as VIX (equity) or GVX (gold), confirms that cryptocurrency volatility dynamics are often disconnected from traditional markets, yet, share common shocks.
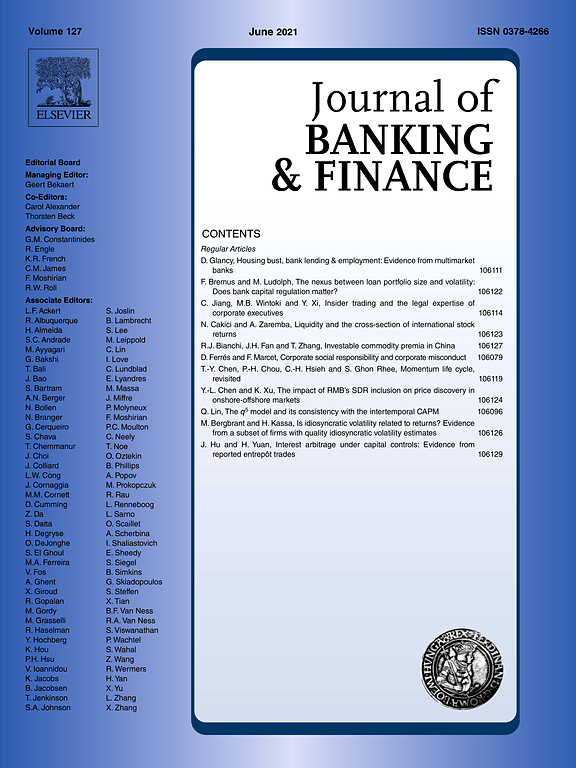
A Factor-model Approach for Correlation Scenarios and Correlation Stress Testing
in: Journal of Banking and Finance, April 2019
Abstract
In 2012, JPMorgan accumulated a USD 6.2 billion loss on a credit derivatives portfolio, the so-called “London Whale”, partly as a consequence of de-correlations of non-perfectly correlated positions that were supposed to hedge each other. Motivated by this case, we devise a factor model for correlations that allows for scenario-based stress testing of correlations. We derive a number of analytical results related to a portfolio of homogeneous assets. Using the concept of Mahalanobis distance, we show how to identify adverse scenarios of correlation risk. In addition, we demonstrate how correlation and volatility stress tests can be combined. As an example, we apply the factor-model approach to the “London Whale” portfolio and determine the value-at-risk impact from correlation changes. Since our findings are particularly relevant for large portfolios, where even small correlation changes can have a large impact, a further application would be to stress test portfolios of central counterparties, which are of systemically relevant size.
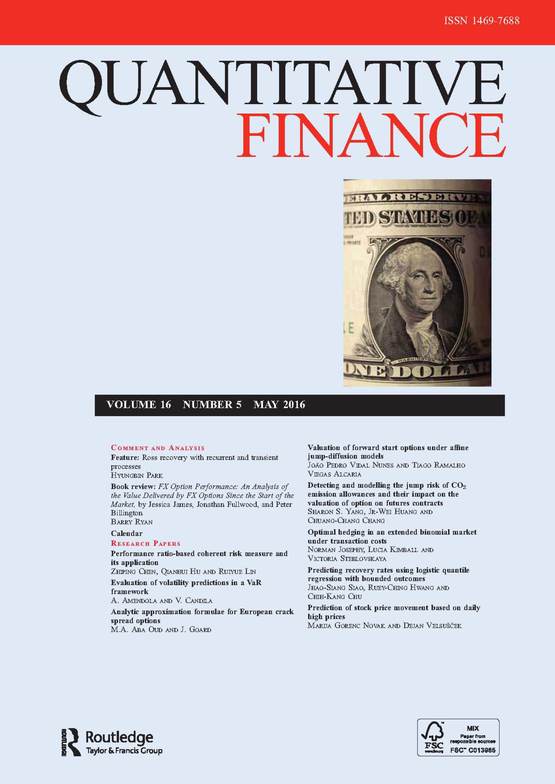
Tail-risk Protection Trading Strategies
in: Quantitative Finance, Nr. 5, 2017
Abstract
Starting from well-known empirical stylized facts of financial time series, we develop dynamic portfolio protection trading strategies based on econometric methods. As a criterion for riskiness, we consider the evolution of the value-at-risk spread from a GARCH model with normal innovations relative to a GARCH model with generalized innovations. These generalized innovations may for example follow a Student t, a generalized hyperbolic, an alpha-stable or a Generalized Pareto distribution (GPD). Our results indicate that the GPD distribution provides the strongest signals for avoiding tail risks. This is not surprising as the GPD distribution arises as a limit of tail behaviour in extreme value theory and therefore is especially suited to deal with tail risks. Out-of-sample backtests on 11 years of DAX futures data, indicate that the dynamic tail-risk protection strategy effectively reduces the tail risk while outperforming traditional portfolio protection strategies. The results are further validated by calculating the statistical significance of the results obtained using bootstrap methods. A number of robustness tests including application to other assets further underline the effectiveness of the strategy. Finally, by empirically testing for second-order stochastic dominance, we find that risk averse investors would be willing to pay a positive premium to move from a static buy-and-hold investment in the DAX future to the tail-risk protection strategy.
Arbeitspapiere
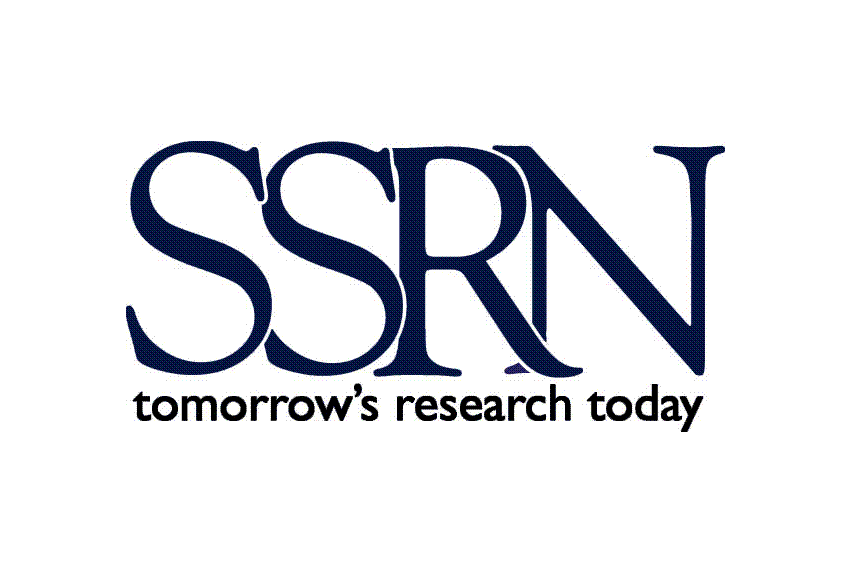
How to Talk Down Your Stock Performance
in: SSRN Discussion Papers, 2020
Abstract
We process the natural language of verbal firm disclosures in order to study the use of context specific language or jargon and its impact on financial performance. We observe that, within the Q&A of earnings conference calls, managers use less jargon in responses to tougher questions, and after a quarter of bad economic success. Moreover, markets interpret the lack of precise information as a bad signal: we find lower cumulative abnormal returns and a higher implied volatility following earnings calls where managers use less jargon. These results support the argument that context specific language or jargon helps to efficiently and precisely transfer information.